导读
由三位图灵奖得主约书亚·本吉奥(yoshua bengio)、杰佛瑞·辛顿(geoffrey hinton)、姚期智(andrew yao)(清华大学人工智能国际治理研究院学术委员会主席)领衔,连同多位权威专家,包括诺贝尔经济学奖得主丹尼尔·卡内曼 (daniel kahneman) 以及清华大学讲席教授张亚勤(清华大学人工智能国际治理研究院学术委员)、文科资深教授薛澜(清华大学苏世民书院院长、公共管理学院学术委员会主任、清华大学人工智能国际治理研究院院长)等共同撰写的文章 “managing extreme ai risks amid rapid progress”(《人工智能飞速进步背景下的极端风险管理》) 于2024年5月20日发表于美国《科学》杂志。
现将全文进行翻译,以飨读者。
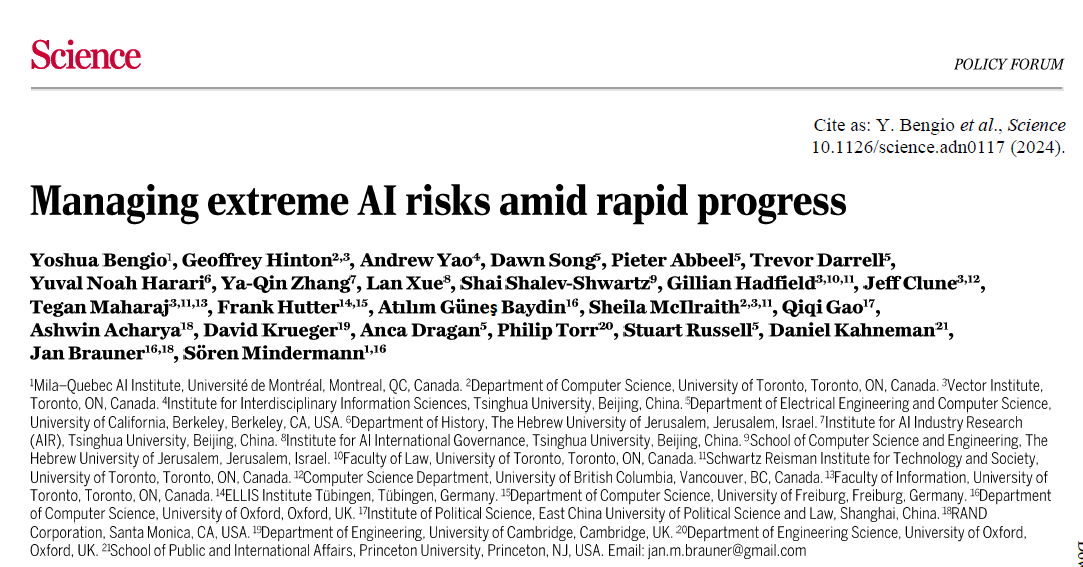
文章摘要
artificial intelligence (ai) is progressing rapidly, and companies are shifting their focus to developing generalist ai systems that can autonomously act and pursue goals. increases in capabilities and autonomy may soon massively amplify ai’s impact, with risks that include large-scale social harms, malicious uses, and an irreversible loss of human control over autonomous ai systems. although researchers have warned of extreme risks from ai [1], there is a lack of consensus about how to manage them. society’s response, despite promising first steps, is incommensurate with the possibility of rapid, transformative progress that is expected by many experts. ai safety research is lagging. present governance initiatives lack the mechanisms and institutions to prevent misuse and recklessness and barely address autonomous systems. drawing on lessons learned from other safety-critical technologies, we outline a comprehensive plan that combines technical research and development (r&d) with proactive, adaptive governance mechanisms for a more commensurate preparation.
在人工智能(ai)快速发展之时,众多企业逐渐将重点转移至开发可自主行动和追求目标的通用ai系统。随着能力与自主性的提高,ai的影响将会被大幅扩大,带来大规模社会危害、恶意使用以及人类不可逆地失去对自主ai系统的控制等风险。尽管研究人员都对ai的极端风险作出了警告,但对于如何管理这些风险依然缺乏共识。尽管在应对措施方面已经有了一些初步进展,但与许多专家所预测且极有可能出现的发展速度和变化程度相比,当前的社会回应仍显不足。ai安全研究已经滞后了。目前的治理举措缺少能预防滥用和使用不当的机制和机构,且几乎没涉及自主系统。鉴于其他关键技术在安全上的经验教训,我们提出了一项将技术研发与主动、适应的治理机制相结合的综合方案,以此来做好更相符的准备。
01 rapid progress, high stakes
快速发展,高风险
present deep-learning systems still lack important capabilities, and we do not know how long it will take to develop them. however, companies are engaged in a race to create generalist ai systems that match or exceed human abilities in most cognitive work. they are rapidly deploying more resources and developing new techniques to increase ai capabilities, with investment in training state-of-the-art models tripling annually.
我们无法精准预计,当前深度学习系统所欠缺的重要能力还需要多长时间才能被开发出来。但进度显然已被加快,因为各公司都在竞相创造在大多数认知工作中匹配或超越人类能力的通用ai系统。他们正在快速地部署更多资源和开发新技术来提高ai能力,每年训练最先进模型的投资已经增加了两倍。
there is much room for further advances because tech companies have the cash reserves needed to scale the latest training runs by multiples of 100 to 1000. hardware and algorithms will also improve: ai computing chips have been getting 1.4 times more cost-effective, and ai training algorithms 2.5 times more efficient, each year. progress in ai also enables faster ai progress — ai assistants are increasingly used to automate programming, data collection, and chip design.
ai得到进一步发展的空间是非常巨大的,因为科技公司拥有的现金储备足以将当前最先进的训练规模再扩大100到1000倍。硬件和算法也将得到改进:ai计算芯片的成本效益每年将提高1.4倍,ai训练算法的效率每年将提高2.5倍。ai的进步也会促进自身的发展速度——ai助手越来越多地用于自动化编程、数据收集和芯片设计。
there is no fundamental reason for ai progress to slow or halt at human-level abilities. indeed, ai has already surpassed human abilities in narrow domains such as playing strategy games and predicting how proteins fold. compared with humans, ai systems can act faster, absorb more knowledge, and communicate at a higher bandwidth. additionally, they can be scaled to use immense computational resources and can be replicated by the millions.
没有证据表明,当ai具有和人类同等水平时,其发展速度就会减缓或停止。事实上,ai已经在玩策略游戏和预测蛋白质如何折叠等狭窄领域超越了人类的能力。与人类相比,ai系统可以更快地行动、吸收更多的知识并以更高的带宽进行通信。此外,它们可以被不断扩展从而使用巨大的计算资源并进行数百万次复制。
we do not know for certain how the future of ai will unfold. however, we must take seriously the possibility that highly powerful generalist ai systems that outperform human abilities across many critical domains will be developed within this decade or the next. what happens then? more capable ai systems have larger impacts. especially as ai matches and surpasses human workers in capabilities and cost-effectiveness, we expect a massive increase in ai deployment, opportunities, and risks. if managed carefully and distributed fairly, ai could help humanity cure diseases, elevate living standards, and protect ecosystems. the opportunities are immense.
我们不确定ai的未来将如何发展。然而,我们必须认真对待这样一种可能性:在未来十年或二十年内,许多在关键领域超越人类能力的强大通用ai系统将会被开发出来。那时会发生什么?能力更强的ai系统会产生更大的影响。特别是当ai在能力和成本效益方面赶上甚至超过人类工作者时,我们预计ai的部署、机遇和风险都将大幅增加。如果管理得当且分配公平,ai也可以帮助人类治愈疾病、提高生活水平、保护生态系统。因此,机遇是巨大的。
but alongside advanced ai capabilities come large-scale risks. ai systems threaten to amplify social injustice, erode social stability, enable large-scale criminal activity, and facilitate automated warfare, customized mass manipulation, and pervasive surveillance.
但先进的ai能力也会带来大规模风险。ai系统会加剧社会不公正,破坏社会稳定,助长大规模犯罪活动,并促进自动化战争、定制化的大规模操纵和无处不在的监视。
many risks could soon be amplified, and new risks created, as companies work to develop autonomous ai: systems that can use tools such as computers to act in the world and pursue goals. malicious actors could deliberately embed undesirable goals. without r&d breakthroughs (see next section), even well-meaning developers may inadvertently create ai systems that pursue unintended goals: the reward signal used to train ai systems usually fails to fully capture the intended objectives, leading to ai systems that pursue the literal specification rather than the intended outcome. additionally, the training data never captures all relevant situations, leading to ai systems that pursue undesirable goals in new situations encountered after training.
各公司致力于开发自主ai的同时,许多风险可能很快会被放大并产生新的风险:这些系统可以利用计算机等工具在全球行动并追求目标。恶意行为者可能会故意嵌入不良目标。如果没有研发突破(见下一节),即使是善意的开发人员也可能会无意中创造追求非预定目标的ai系统:用于训练ai系统的奖励信号通常无法完全指向预定目标,导致ai系统追求字面规范而非预期结果。此外,训练数据永远无法涵盖所有情况,导致ai系统在训练后遇到新情况时导向不良目标。
once autonomous ai systems pursue undesirable goals, we may be unable to keep them in check. control of software is an old and unsolved problem: computer worms have long been able to proliferate and avoid detection. however, ai is making progress in critical domains such as hacking, social manipulation, and strategic planning and may soon pose unprecedented control challenges. to advance undesirable goals, ai systems could gain human trust, acquire resources, and influence key decision-makers. to avoid human intervention, they might copy their algorithms across global server networks. in open conflict, ai systems could autonomously deploy a variety of weapons, including biological ones. ai systems having access to such technology would merely continue existing trends to automate military activity. finally, ai systems will not need to plot for influence if it is freely handed over. companies, governments, and militaries may let autonomous ai systems assume critical societal roles in the name of efficiency.
一旦自主ai系统导向不良目标,我们可能无法控制它们。软件控制是一个久远且尚未解决的问题:计算机蠕虫长期以来一直能够扩散并躲避检测。然而,ai正在黑客攻击、社交操纵和战略规划等关键领域取得进展,可能很快会带来前所未有的控制挑战。为了推进不良目标,ai系统可能会取得人类信任、获取资源并影响关键决策者。为了避免人为干预,它们可能会在全球服务器网络上复制其算法。在公开冲突中,ai系统可能会自主部署各种武器,包括生物武器。拥有此类技术的ai系统只会延续已有的军事活动自动化趋势。最后,如果ai系统被很轻易地赋予关键的社会角色,它们将不再需要阴谋来获得影响力。公司、政府和军队都有可能会以效率的名义让自主ai系统承担关键的社会角色。
without sufficient caution, we may irreversibly lose control of autonomous ai systems, rendering human intervention ineffective. large-scale cybercrime, social manipulation, and other harms could escalate rapidly. this unchecked ai advancement could culminate in a large scale loss of life and biosphere, and the marginalization or extinction of humanity.
如果缺乏足够的谨慎,我们可能会不可逆转地失去对自主ai系统的控制,导致人类的干预无效。大规模网络犯罪、社交操纵和其他危害可能会迅速升级。这种不受控制的ai进展可能最终导致生命和生物圈的大规模损失,以及人类被边缘化或灭绝。
we are not on track to handle these risks well. humanity is pouring vast resources into making ai systems more powerful but far less into their safety and mitigating their harms. only an estimated 1 to 3% of ai publications are on safety. for ai to be a boon, we must reorient; pushing ai capabilities alone is not enough.
我们还没做好应对这些风险的准备。人类正在投入大量资源,使ai系统变得更强大,但对其安全性和减轻危害方面的投入却投入甚少。据估计,只有1%到3%关于ai的出版物是讲安全的。为了让ai造福人类,我们必须重新调整,仅仅推动ai能力发展是不够的。
we are already behind schedule for this reorientation. the scale of the risks means that we need to be proactive, because the costs of being unprepared far outweigh those of premature preparation. we must anticipate the amplification of ongoing harms, as well as new risks, and prepare for the largest risks well before they materialize.
在重新调整的进度中,我们依然处于落后状态。大规模风险意味着我们要更积极主动,毫无准备的代价远远大于提早准备的代价。我们必须预见到当前危害的进一步扩大以及新风险出现,并在最大风险发生之前做好准备。
02 reorient technical r&d
重新调整技术研发
there are many open technical challenges in ensuring the safety and ethical use of generalist, autonomous ai systems. unlike advancing ai capabilities, these challenges cannot be addressed by simply using more computing power to train bigger models. they are unlikely to resolve automatically as ai systems get more capable and require dedicated research and engineering efforts. in some cases, leaps of progress may be needed; we thus do not know whether technical work can fundamentally solve these challenges in time. however, there has been comparatively little work on many of these challenges. more r&d may thus facilitate progress and reduce risks. a first set of r&d areas needs breakthroughs to enable reliably safe ai. without this progress, developers must either risk creating unsafe systems or falling behind competitors who are willing to take more risks. if ensuring safety remains too difficult, extreme governance measures would be needed to prevent corner-cutting driven by competition and overconfidence. these r&d challenges include the following:
在确保通用、自主ai系统的安全和道德使用方面,存在许多公开的技术挑战。与提高ai能力不同的是,这些挑战不能只通过使用更多算力培训更大模型来解决。这些挑战也不可能会随着ai系统能力的提高而自动解决,反而需要更有针对性的研究和工程开发。在某些情况下,可能需要突破性进展;因此,我们不知道技术发展能否及时从根本上解决这些挑战。针对这些挑战而开展的工作相对较少。我们需要更多能促进发展和降低风险的研发。第一组需要实现突破的研发领域是实现可靠安全的ai。否则,开发人员如果不冒着创建不安全系统的风险,那么就会落后于更冒进的竞争对手。如果确保安全还是太难实现,那就需要采取一些极端的治理措施,以防止因竞争和过度自信导致的“偷工减料”。这些研发挑战包括以下内容:
oversight and honesty more capable ai systems can better exploit weaknesses in technical oversight and testing, for example, by producing false but compelling output.
监督与诚信 能力更强的ai系统将会更好地利用技术监督和测试方面的缺陷,例如,生产虚假但令人信服的输出。
robustness ai systems behave unpredictably in new situations. whereas some aspects of robustness improve with model scale, other aspects do not or even get worse.
鲁棒性 ai系统在新情况下的表现难以预测。鲁棒性的某些方面会随着模型规模而改善,而其他方面则不会,甚至会变得更糟。
interpretability and transparency ai decision-making is opaque, with larger, more capable models being more complex to interpret. so far, we can only test large models through trial and error. we need to learn to understand their inner workings.
可解释性和透明度 ai决策是不透明的,规模更大、能力更强的模型就更难以解释。到目前为止,我们只能通过试错来测试大模型。我们需要学会去理解它们的内部运作机制。
inclusive ai development ai advancement will need methods to mitigate biases and integrate the values of the many populations it will affect.
包容的ai发展 发展ai需要用各种方法来减轻偏见,并整合将其会影响的众多群体的价值观。
addressing emerging challenges future ai systems may exhibit failure modes that we have so far seen only in theory or lab experiments, such as ai systems taking control over the training reward-provision channels or exploiting weaknesses in our safety objectives and shutdown mechanisms to advance a particular goal.
应对新兴挑战 未来的ai系统可能会表现出迄今为止我们仅在理论或实验室实验中看到过的失效模式,例如ai系统掌控“训练奖励—供应”渠道,或利用我们在安全目标和关闭机制中的缺陷来推进某一特定目标。
a second set of r&d challenges needs progress to enable effective, risk-adjusted governance or to reduce harms when safety and governance fail.
第二组需要取得进展的研发领域是,实现有效的、风险调整的治理,或在安全措施和治理失效时减少危害。
evaluation for dangerous capabilities as ai developers scale their systems, unforeseen capabilities appear spontaneously, without explicit programming. they are often only discovered after deployment. we need rigorous methods to elicit and assess ai capabilities and to predict them before training. this includes both generic capabilities to achieve ambitious goals in the world (e.g., long-term planning and execution) as well as specific dangerous capabilities based on threat models (e.g., social manipulation or hacking). present evaluations of frontier ai models for dangerous capabilities, which are key to various ai policy frameworks, are limited to spot-checks and attempted demonstrations in specific settings. these evaluations can sometimes demonstrate dangerous capabilities but cannot reliably rule them out: ai systems that lacked certain capabilities in the tests may well demonstrate them in slightly different settings or with post training enhancements. decisions that depend on ai systems not crossing any red lines thus need large safety margins. improved evaluation tools decrease the chance of missing dangerous capabilities, allowing for smaller margins.
评估危险能力随着ai开发人员对系统进行扩展,不可预见的能力会在没有明确编程的情况下自发出现,通常只有在部署后才会被发现。我们需要严格的方法来探知和评估ai能力,并在训练前对其进行预测。这既包括在世界上实现宏伟目标的通用能力(如长期规划和执行),也包括基于威胁模型的特定危险能力(如社交操纵或黑客攻击)。目前对前沿ai模型危险能力的评估——仅限于在特定环境下的抽查和的推演——是各种ai政策框架的关键。这些评估有时可以展示危险能力,但不能完全排除它们:在测试中缺乏某些能力的ai系统,很可能在稍有不同的环境下或经过后期训练增强后显示出这些能力。因此,在ai系统不跨越任何红线基础上的决策需要更大的安全边界。改进的评估工具可以降低遗漏危险能力的几率,从而允许更小的安全边界。
evaluating ai alignment if ai progress continues, ai systems will eventually possess highly dangerous capabilities. before training and deploying such systems, we need methods to assess their propensity to use these capabilities. purely behavioral evaluations may fail for advanced ai systems: similar to humans, they might behave differently under evaluation, faking alignment.
评估人工智能对齐 如果ai继续发展下去,ai系统最终将拥有高度危险的能力。在训练和部署这些系统之前,我们需要一些方法来评估系统使用这些能力的倾向。对于先进的ai系统,单纯的行为评估可能会失败:与人类类似,它们可能会在评估中刻意表现不同,从而制造虚假对齐。
risk assessment we must learn to assess not just dangerous capabilities but also risk in a societal context, with complex interactions and vulnerabilities. rigorous risk assessment for frontier ai systems remains an open challenge owing to their broad capabilities and pervasive deployment across diverse application areas.
风险评估 我们不仅要学会评估ai产生的直接风险,还要学会评估具有复杂性和脆弱性的社会背景下ai产生的一系列风险。事实上,鉴于前沿ai系统具有通用性能力,被广泛应用于众多领域,对相关系统进行严格的风险评估仍然是一项重要挑战。
resilience inevitably, some will misuse or act recklessly with ai. we need tools to detect and defend against ai-enabled threats such as large-scale influence operations, biological risks, and cyberattacks. however, as ai systems become more capable, they will eventually be able to circumvent humanmade defenses. to enable more powerful ai based defenses, we first need to learn how to make ai systems safe and aligned.
韧性不可避免的是,有些人会滥用、恶用ai。我们需要各类工具以检测和防御ai赋能产生的威胁,如大规模影响力行动、生物风险、网络攻击等。随着ai系统的能力越来越强,它们规避人为防御的能力也在不断增强。为了实现更强大的基于ai的防御,我们首先需要学习如何确保ai系统的安全和对齐。
given the stakes, we call on major tech companies and public funders to allocate at least one-third of their ai r&d budget, comparable to their funding for ai capabilities, toward addressing the above r&d challenges and ensuring ai safety and ethical use. beyond traditional research grants, government support could include prizes, advance market commitments, and other incentives. addressing these challenges, with an eye toward powerful future systems, must become central to our field.
鉴于事关重大,我们呼吁大型科技公司和公共资助者至少将其ai研发预算的三分之一用于解决上述挑战并确保ai安全和道德使用。除了传统的研发投入外,政府还可以提供奖金、预先市场承诺等各类激励措施。着眼于强大的未来ai系统,应对这些挑战必须成为我们关注的核心。
03 governance measures
治理措施
we urgently need national institutions and international governance to enforce standards that prevent recklessness and misuse. many areas of technology, from pharmaceuticals to financial systems and nuclear energy, show that society requires and effectively uses government oversight to reduce risks. however, governance frameworks for ai are far less developed and lag behind rapid technological progress. we can take inspiration from the governance of other safety-critical technologies while keeping the distinctiveness of advanced ai in mind—that it far outstrips other technologies in its potential to act and develop ideas autonomously, progress explosively, behave in an adversarial manner, and cause irreversible damage. governments worldwide have taken positive steps on frontier ai, with key players, including china, the united states, the european union, and the united kingdom, engaging in discussions and introducing initial guidelines or regulations. despite their limitations—often voluntary adherence, limited geographic scope, and exclusion of high-risk areas like military and r&d-stage systems—these are important initial steps toward, among others, developer accountability, third-party audits, and industry standards.
我们迫切需要国家机构和国际治理来执行ai误用和和滥用的预防标准。生物医药、金融、到核能等众多技术领域的经验表明,社会需要通过政府监督降低风险。然而,ai的治理框架远远落后于技术的快速发展。我们可以从其他高风险技术的治理中汲取灵感,同时牢记前沿ai技术的独特性——ai在自主行动和自主意识、对抗性行为及造成不可逆损害等方面远远超过其他技术。目前,包括中国、美国、欧盟和英国等主要参与者在前沿ai上采取了积极举措,提出了初步指导方针或法规。尽管这些方针或法规存在以自愿遵守为主、地理范围有限、不包括军事等高风险领域等局限性,但仍朝着开发者问责制、第三方监管的行业标准等治理方向迈出了重要的第一步。
yet these governance plans fall critically short in view of the rapid progress in ai capabilities. we need governance measures that prepare us for sudden ai breakthroughs while being politically feasible despite disagreement and uncertainty about ai timelines. the key is policies that automatically trigger when ai hits certain capability milestones. if ai advances rapidly, strict requirements automatically take effect, but if progress slows, the requirements relax accordingly. rapid, unpredictable progress also means that risk-reduction efforts must be proactive—identifying risks from next generation systems and requiring developers to address them before taking high-risk actions. we need fast-acting, tech savvy institutions for ai oversight, mandatory and much-more rigorous risk assessments with enforceable consequences (including assessments that put the burden of proof on ai developers), and mitigation standards commensurate to powerful autonomous ai. without these, companies, militaries, and governments may seek a competitive edge by pushing ai capabilities to new heights while cutting corners on safety or by delegating key societal roles to autonomous ai systems with insufficient human oversight, reaping the rewards of ai development while leaving society to deal with the consequences.
鉴于ai能力的快速进步,上述治理计划远远不够。尽管目前各届关于ai发展的时间表仍有分歧,但人类仍需采取政治上可行的方式,为ai领域随时可能产生的技术突破做好准备。实现这一目标的关键就是提前设定ai达到某些能力阈值时自动触发的监管政策,即如果ai发展迅速,严格的监管要求就会自动生效,但如果ai发展缓慢,监管政策就会相应放宽。同时,ai快速、不可预测的发展还意味着要人类需要提前识别下一代ai系统的潜在风险,并要求相关系统的开发人员提前准备控制风险的相关措施。最后,我们还需要行动迅速、精通技术的行政机构来监督ai,需要强制性的、更加严格的风险评估及执行措施(包括要求ai开发者承担举证责任)。如果没有上述措施,公司、军队和政府可能会为了寻求竞争优势而将ai能力盲目推向新的高度,但在安全问题上“偷工减料”;或将关键的社会角色委托给自主ai系统,却没有提供足够的人类监督,让全社会承担ai系统可能带来的负面影响。
institutions to govern the rapidly moving frontier of ai to keep up with rapid progress and avoid quickly outdated, inflexible laws national institutions need strong technical expertise and the authority to act swiftly. to facilitate technically demanding risk assessments and mitigations, they will require far greater funding and talent than they are due to receive under almost any present policy plan. to address international race dynamics, they need the affordance to facilitate international agreements and partnerships. institutions should protect low-risk use and low-risk academic research by avoiding undue bureaucratic hurdles for small, predictable ai models. the most pressing scrutiny should be on ai systems at the frontier: the few most powerful systems, trained on billion-dollar supercomputers, that will have the most hazardous and unpredictable capabilities.
应对人工智能前沿快速发展的治理机构为了跟上ai技术快速发展的步伐,避免治理体系的相对落后,国家机构需要强大的技术能力和迅速采取行动的权力。为了实现高要求的技术风险评估和治理,这些机构需要远超现行行政机构的资金和人才。为了应对ai领域的国际竞争,这些机构还需要具备推动国际协议和伙伴关系的对外交流能力。同时,这些机构需要避免针对小型、可预测的ai模型设置不当的官僚障碍,保护低风险领域ai技术的使用和学术研究。目前最迫切的监管需求还是集中在少数能力最强、最前沿的,具有危险的不可预测能力的ai系统。
government insight to identify risks, governments urgently need comprehensive insight into ai development. regulators should mandate whistleblower protections, incident reporting, registration of key information on frontier ai systems and their datasets throughout their life cycle, and monitoring of model development and supercomputer usage. recent policy developments should not stop at requiring that companies report the results of voluntary or underspecified model evaluations shortly before deployment. regulators can and should require that frontier ai developers grant external auditors on-site, comprehensive (“white box”), and fine-tuning access from the start of model development. this is needed to identify dangerous model capabilities such as autonomous self-replication, large scale persuasion, breaking into computer systems, developing (autonomous) weapons, or making pandemic pathogens widely accessible.
政府洞察力 为了识别风险,政府迫切需要全面了解ai的发展情况。监管机构应强制记录前沿ai系统及其整个生命周期数据集的关键信息,监控相关模型的开发和超级计算机的使用。最新的政策发展不应局限于要求公司在部署前才报告模型评估结果,监管机构可以要求前沿ai开发者从模型开发伊始就授予外部人员审查、“白盒”和微调的访问权限。这些监管措施对于识别自主自我复制、大规模说服、侵入计算机系统、开发(自主)武器或使流行病病原体广泛传播等风险是极为必要的。
safety cases despite evaluations, we cannot consider coming powerful frontier ai systems “safe unless proven unsafe.” with present testing methodologies, issues can easily be missed. additionally, it is unclear whether governments can quickly build the immense expertise needed for reliable technical evaluations of ai capabilities and societal-scale risks. given this, developers of frontier ai should carry the burden of proof to demonstrate that their plans keep risks within acceptable limits. by doing so, they would follow best practices for risk management from industries, such as aviation, medical devices, and defense software, in which companies make safety cases: structured arguments with falsifiable claims supported by evidence that identify potential hazards, describe mitigations, show that systems will not cross certain red lines, and model possible outcomes to assess risk. safety cases could leverage developers’ in-depth experience with their own systems. safety cases are politically viable even when people disagree on how advanced ai will become because it is easier to demonstrate that a system is safe when its capabilities are limited. governments are not passive recipients of safety cases: they set risk thresholds, codify best practices, employ experts and third-party auditors to assess safety cases and conduct independent model evaluations, and hold developers liable if their safety claims are later falsified.
安全论证 哪怕按照上述步骤进行评估,我们仍然无法将即将到来的强大前沿ai系统视为“在未证明其不安全之前就是安全的”。使用现有的测试方法,很容易出现遗漏问题。此外,我们尚不清楚政府能否迅速建立对ai能力和社会规模风险进行可靠技术评估所需的大量专业知识。有鉴于此,前沿ai的开发者应该承担相应责任,证明他们的ai模型将风险控制在可接受的范围内。通过多方参与,开发者们将遵循航空、医疗设备、国防软件等行业风险管理的历史实践。在上述行业中,公司被要求提出安全案例,通过结构化的论证、可证伪的分析和情景模识别潜在风险、划清红线,这一模式可以充分利用开发人员对相关系统的深入了解。同时,即使人们对ai的先进程度存在分歧,安全案例在政治上也是可行的,因为在ai系统能力有限的情况下,证明系统是安全反而更加容易。最后,政府并不是安全论证的被动接受者,而是可以通过设置风险阈值、制定最佳实践规范、聘请专家和第三方机构评估安全论证等形式进行管理,并在开发者安全声明被证伪时追究其责任。
mitigation to keep ai risks within acceptable limits, we need governance mechanisms that are matched to the magnitude of the risks. regulators should clarify legal responsibilities that arise from existing liability frameworks and hold frontier ai developers and owners legally accountable for harms from their models that can be reasonably foreseen and prevented, including harms that foreseeably arise from deploying powerful ai systems whose behavior they cannot predict. liability, together with consequential evaluations and safety cases, can prevent harm and create much-needed incentives to invest in safety.
缓解措施 为了将ai风险控制在可接受的范围内,我们需要建立与风险等级相匹配的治理机制。监管机构应明确现有责任框架划定的法律责任,并要求前沿ai系统的开发者和所有者对其模型所产生的、可以合理预见和预防的危害承担法律责任,包括可以预见的源于部署强大ai系统(其行为无法预测)造成的损害。责任与后果评估应该和安全论证一起,为ai风险治理提供保障。
commensurate mitigations are needed for exceptionally capable future ai systems, such as autonomous systems that could circumvent human control. governments must be prepared to license their development, restrict their autonomy in key societal roles, halt their development and deployment in response to worrying capabilities, mandate access controls, and require information security measures robust to state-level hackers until adequate protections are ready. governments should build these capacities now.
针对能力超强的未来ai系统,例如能够绕过人类控制的自主系统,我们需要采取相应的缓解措施。政府必须做好准备,对这类系统的开发进行管理,限制其在关键社会角色中的自主性,并针对令人担忧的能力暂停其研发和部署。同时,政府应强制实施访问控制,要求其具备抵御国家级黑客的安全措施。政府应从现在开始着手建立这些能力。
to bridge the time until regulations are complete, major ai companies should promptly lay out “if-then” commitments: specific safety measures they will take if specific red-line capabilities are found in their ai systems. these commitments should be detailed and independently scrutinized. regulators should encourage a race-to-the top among companies by using the best-in class commitments, together with other inputs, to inform standards that apply to all players.
为了缩短监管完善的空窗期,主要的ai公司应该迅速作出“if-then”的承诺,即如果在他们的 ai 系统中发现了特定的越界能力,他们将采取针对性的安全措施。这些承诺应该足够详细且经过独立审查。监管机构应鼓励公司之间进行“向上看齐”的竞争,利用同类最佳的承诺制定适用于所有参与者的共同标准。
to steer ai toward positive outcomes and away from catastrophe, we need to reorient. there is a responsible path—if we have the wisdom to take it.
为了引导人工智能“智能向善”并避免灾难性后果,我们需要及时调整治理方向。只要我们有足够智慧,一定能够找到一条实现“负责任的人工智能”的道路。
供稿丨清华大学人工智能国际治理研究院
清华大学应急管理研究基地